(Only some are more equal than others)
Today we introduce you to one of the Jisc-funded PhD. students working at The Knowledge Media Institute (KMi), which is a part of the Open University and is located in Milton Keynes. David Pride is one of the team working as a part of the joint Jisc/OU CORE project (COnnecting REpositores) which offers Open Access to over eight million research papers.
David Pride completed his MSc. in Computer Science (with distinction) at The University of Hertfordshire in 2016 before starting his PhD. at KMi in February of this year. David’s PhD. supervisor is Dr. Petr Knoth and his thesis topic is looking at web-scale research analytics for identifying high performance and trends in academic research. In short, this involves using state-of-the-art Text and Data Mining techniques to analyse datasets containing millions of academic papers to attempt to identify highly impactful and influential research.
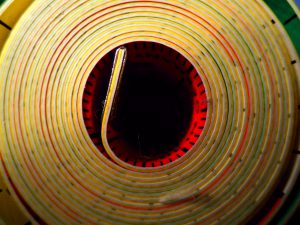
http://bit.ly/2AilG21 *
At KMi, all PhD. students must complete a pilot project study within their first year. For his, David chose to undertake a review of several previous studies that have attempted to automatically categorise citations according to type, sentiment and influence. Current bibliometrics methods, from the renowned Journal Impact Factor (JIF) to the h-index for individual authors, treat all citations equally. There is much empirical evidence demonstrating that treating citations all equally in this manner means that basic citation counts do not reflect the true picture of how a paper may be being used. A piece of research may be highly cited because of its ground-breaking content or because it introduces a new methodology. However, it could also be highly cited because it is a survey paper that provides a rich background to a particular domain. Conversely, a paper may engender citations that refute or disagree with the original work. Whilst most citations are overtly neutral in sentiment there is a certain percentage of negative citations. Yet, currently, all these citations are treated equally.
David’s work is also focused on developing new metrics that can leverage the full content of an academic paper to evaluate its quality rather than relying on citation counts alone. He therefore continued the work of previous studies in using machine learning and natural language processing tools to automatically classify citations according to type and ‘influence’. Influence itself is an interesting concept and, in this case, refers to how influential the cited paper was on the citing paper, i.e. was the citation central to understanding the new work or was it perfunctory, or merely mentioned as part of the literature review for example. If information regarding how a paper is being cited is available to academics, researchers and reviewers this provides a much richer insight than currently available with basic citation counts.
Building on the work of Valenzuela et al. (2015) and Zhu et al. (2015) David developed a system to classify citations in a paper as either incidental or influential. Despite running into several difficult steps along the way, the results of the experiments were overall extremely positive and the resulting short paper was presented at the TPDL (Theory and Practice of Digital Libraries) 2017 Conference and was published in the Springer Lecture Notes on Computer Science. A full version of the paper was later accepted to the ISSI (International Society of Scientometrics and Informetrics (2017) where David presented his results to conference in Wuhan, China.
Moving forward, David intends to address one of the major failings in this domain which is the lack of a massive scale human-annotated dataset of citations to use when training classifiers for this task. It is believed that the results obtained previously can be significantly improved with a larger initial training set. Citation data is unbalanced in nature, negative citations for example representing only about 4% of all citations. Training a classifier to accurately identify these citations requires a dataset of sufficient magnitude to contain enough examples of every class. A large-scale reference set which contains citations annotated according to type, sentiment and influence would be an extremely valuable asset for researchers working in this domain.
In the coming months, David will also be researching the peer review process and how well this correlates with current methodologies for tracking research excellence. He has some interesting data he is currently looking at and we’re looking forward to seeing what he produces in 2018!
*Featured image: “measurement” by flui., used under the terms of a Creative Commons Attribution license.